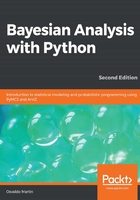
Programming Probabilistically
Now that we have a basic understanding of Bayesian statistics, we are going to learn how to build probabilistic models using computational tools. Specifically, we are going to learn about probabilistic programming with PyMC3. The basic idea is to specify models using code and then solve them in a more or less automatic way. It is not that we are too lazy to learn the mathematical way, nor are we elitist-hardcore-hackers-in-code. One important reason behind this choice is that many models do not lead to an analytic closed form, and thus we can only solve those models using numerical techniques.
Another reason to learn probabilistic programming is that modern Bayesian statistics is mainly done by writing code, and since we already know Python, why would we do it in another way? Probabilistic programming offers an effective way to build and solve complex models and allows us to focus more on model design, evaluation, and interpretation, and less on mathematical or computational details. In this chapter, and through the rest of this book, we are going to use PyMC3, a very flexible Python library for probabilistic programming, as well as ArviZ, a new Python library that will help us interpret the results of probabilistic models. Knowing PyMC3 and ArviZ will also help us to learn advanced Bayesian concepts in a more practical way.
In this chapter, we will cover the following topics:
- Probabilistic programming
- PyMC3 primer
- The coin-flipping problem revisited
- Summarizing the posterior
- The Gaussian and student's t models
- Comparing groups and the effect size
- Hierarchical models and shrinkage