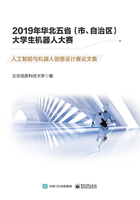
基于MobileNetv 2回归网络算法的无创血糖预测系统研究
贺梦嘉1,吴迎年1,李新1,曹荣敏1,杨桂明1
1. 北京信息科技大学,北京市,100192
摘要:目前有创血糖检测会造成强烈的不适感和感染风险,故无创血糖预测的研究具有很强的现实意义和科研价值。最热门的光学方法设备笨重,不便于实际使用,本文采用红外线热像图来进行血糖的预测,属于人脸工程的范畴。深度学习算法提取特征较多、计算速度慢是阻碍其实用化的关键问题,本文采用轻量化网络MobileNetv 2回归网络对红外线热像图进行回归建模,MobileNetv 2回归网络用深度可分卷积和倒残差块进行速度提升,它便于移植到嵌入式设备、手机端和各种云端接口,MobileNetv 2回归网络在预测集上的速度和预测效果都比较理想,为以后的无创血糖预测算法的便捷接入研究和检测形式提供了一种新的研究方法和设计思路。
关键词:无创血糖预测;红外线热像图预测;单变量预测;轻量化网络;MobileNetv 2回归网络;人工智能;深度学习;人脸工程;卷积神经网络
Research on non-invasive blood glucose prediction system based on mobilenetv 2 regression network algorithms
He Mengjia1,Wu Yingnian1,Li Xin1,Cao Rongmin1,Yang Guiming1
1.Beijing Information Science and Technology University, Beijing City, 100192
Abstract:At present, invasive blood sugar will cause strong discomfort and infection risk, so the research of non-invasive blood sugar has strong practical significance and scientific research value. The most popular optical method equipment is bulky and not easy to use. This paper uses infrared thermography to predict blood sugar, which belongs to the category of face engineering. Deep learning algorithm extracts more features, and slow calculation speed is the key problem hindering its practicality. In this paper, the lightweight network MobileNetv 2 regression network is used to model the infrared thermal image. MobileNetv 2 regression network uses depth separable convolution and inverse residual blocks to speed up. It is easy to transplant to embedded devices, mobile terminals and various cloud interfaces. MobileNetv 2 regression network has good speed and prediction effect on prediction set. It provides a new research method and design idea for the convenient access research and detection form of non-invasive blood sugar detection algorithm in the future.
Keywords:Non-invasive blood sugar prediction;Infrared thermography prediction;Univariate prediction; Lightweight network; MobileNetv 2 regression network; Artificial intelligence; Deep learning;Face engineering;Convolutional neural network