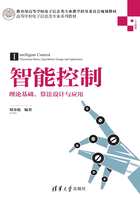
5.1 T-S模糊模型
T-S(Takagi-Sugeno)模糊模型由Takagi和Sugeno两位学者在1985年提出。该模型的主要思想是将非线性系统用许多线段相近地表示出来,即将复杂的非线性问题转化为在不同小线段上的问题。
5.1.1 T-S模糊模型的形式
前面介绍的为传统的模糊系统,属于Mamdani模糊模型,其输出为模糊量。另一种模糊模型为T-S模糊模型,其输出为常量或线性函数,其函数形式为
y=a

T-S模糊模型与Mamdani模糊模型的区别在于,一是T-S模糊模型输出变量为常量或线性函数;二是T-S模糊模型输出为精确量。
T-S型的模糊推理系统非常适合于分段线性控制系统,例如在导弹、飞行器的控制中,可根据高度和速度建立T-S型的模糊推理系统,实现性能良好的线性控制。
5.1.2 仿真实例
设输入X∈[0,5],Y∈[0,10],将它们模糊化为两个模糊量,即“小”和“大”。输出Z为输入(x,y)的线性函数,模糊规则为
If X is small and Y is small then Z =-x+y-3
If X is small and Y is big then Z =x+y+1
If X is big and Y is small then Z =-2y+2
If X is big and Y is big then Z =2x+y-6
仿真程序见chap5_1.m。采用高斯隶属函数对输入进行模糊化,模糊推理系统的输入隶属函数曲线及输入/输出曲线如图5.1和图5.2所示。

图5.1 T-S模糊推理系统的输入隶属函数曲线

图5.2 T-S模糊推理系统的输入/输出曲线
通过命令showrule(ts2)可显示模糊控制规则,共以下4条:
(1)If(X is small)and(Y is small)then(Z is first area)(1)
(2)If(X is small)and(Y is big)then(Z is second area)(1)
(3)If(X is big)and(Y is small)then(Z is third area)(1)
(4)If(X is big)and(Y is big)then(Z is fourth area)(1)
仿真程序:chap5_1.m

5.1.3 一类非线性系统的T-S模糊建模
考虑如下非线性系统[1]

其中,x1(t)∈[-1,1],x2(t)∈[-1,1]。
上式可写为

其中,x(t)=[x1(t) x2(t)]T。
定义

则

考虑x1(t)∈[-1,1],x2(t)∈[-1,1],则


针对z1(t),采用模糊集M1(z1(t))和M2(z1(t))来描述,针对z2(t),采用模糊集N1(z2(t))和N2(z2(t))来描述。采用三角形隶属函数分别描述z1(t)和z2(t)的模糊集,如图5.3和图5.4所示。隶属函数设计为



图5.3 M1(z1(t))和M2(z1(t))隶属函数

图5.4 N1(z2(t))和N2(z2(t))隶属函数
将模糊集模糊化为两个模糊量,即“小”和“大”。模糊规则为
Rule1:If z1(t)is Big and z2(t)is Big then (t)=A1x(t)
Rule2:If z1(t)is Big and z2(t)is Small then (t)=A2 x(t)
Rule3:If z1(t)is Small and z2(t)is Big then (t)=A3 x(t)
Rule4:If z1(t)is Small and z2(t)is Small then (t)=A4 x(t)
结合式(5.4)~式(5.6),可得

模糊T-S模型输出为

其中
h1(z(t))=M1(z1(t))×N1(z2(t))
h2(z(t))=M1(z1(t))×N2(z2(t))
h3(z(t))=M2(z1(t))×N1(z2(t))
h4(z(t))=M2(z1(t))×N2(z2(t))
可见,通过T-S模糊建模,可将非线性系统式(5.2)在x1(t)∈[-1,1],x2(t)∈[-1,1]域内转化为线性系统的形式。
仿真程序:
(1)M1(z1(t))和M2(z1(t))隶属函数:chap5_2.m
%Define N+1 triangle membership function clear all; close all; z1=-1:0.01:1; M1=(z1+1)/2; M2=(1-z1)/2; figure(1); plot(z1,M1); hold on; plot(z1,M2); xlabel('z1)'; ylabel('Degree of membership)';
(2)N1(z2(t))和N2(z2(t))隶属函数:chap5_3.m
%Define N+1 triangle membership function clear all; close all; z2=0:0.01:4; N1=z2/4; N2=(4-z2)/4; figure(1); plot(z2,N1); hold on; plot(z2,N2); xlabel('z2)'; ylabel('Degree of membership)';